Theoretical Calculations
Introduction
In the field of combustion chemistry, the chemical kinetics calculation is related to the maximization of combustion efficiency and energy utilization and directly affects the generation and control of pollutants during the combustion process. As a fundamental science, it provides the key to understanding and predicting the complex chemical changes in the combustion process, which is of great significance for achieving clean and efficient energy conversion.
Our research group is committed to using advanced computational chemistry, molecular simulation techniques and machine learning prediction methods to delve into the microscopic mechanisms of combustion processes. These studies help us understand the basic chemical principles of combustion processes and provide theoretical guidance and technical support for the development of combustion technology.
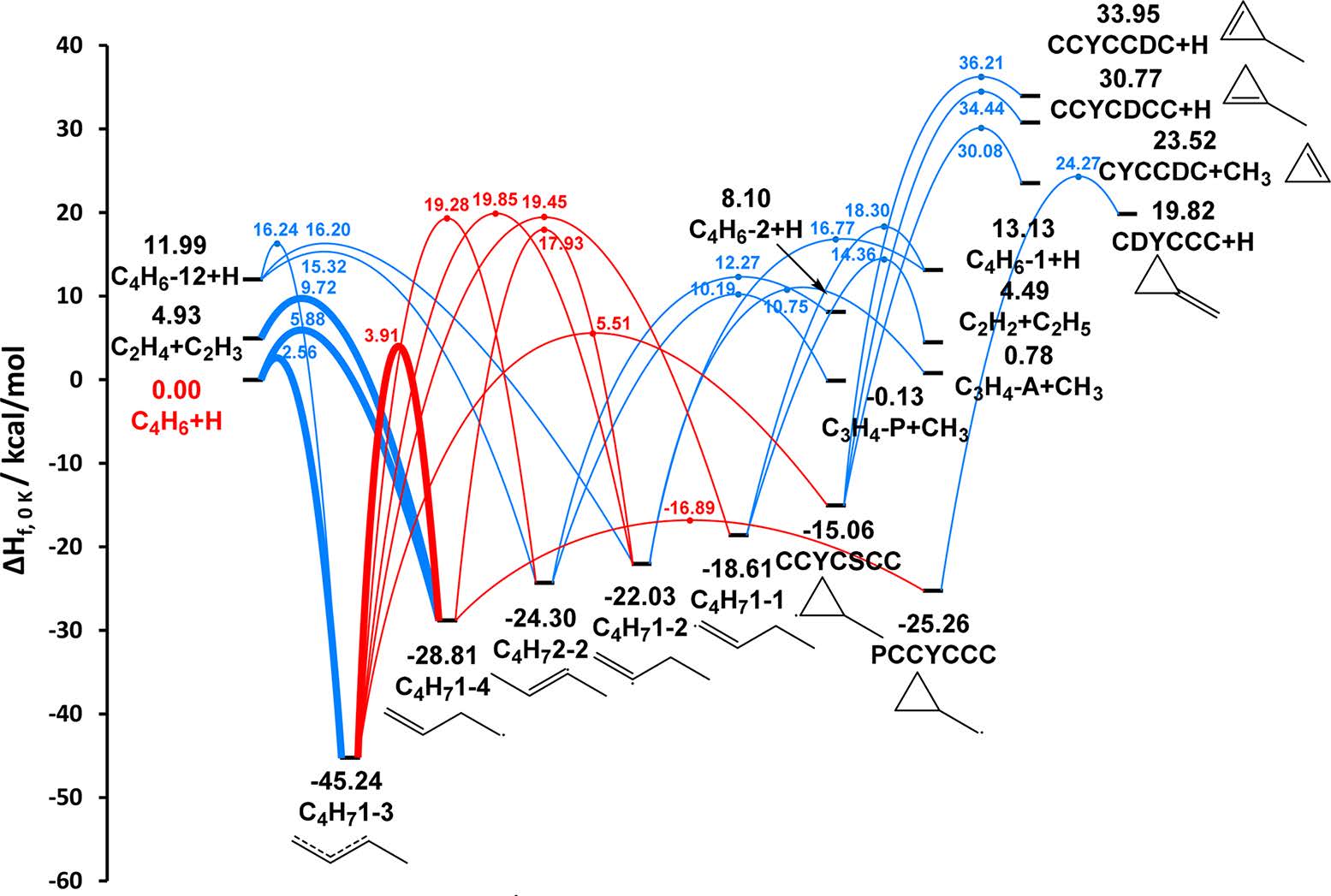
Fig. 1. Ċ4H7 potential-energy surface. (Y. Li, S.J. Klippenstein, C.-W. Zhou, H.J. Curran, The Journal of Physical Chemistry A 121 (2017) 7433-7445.)
Quantum chemical calculations
Our research group utilizes quantum chemical theories and high-level computational methods to characterize the key reactions in the combustion processes of traditional gasoline components, hydrazine-based fuels for liquid rocket engines, nitro compounds, and energetic materials, and to calculate the thermodynamic properties of key species. These data are crucial for understanding the detailed chemical kinetics behavior in combustion processes and provide theoretical and data support for the construction of kinetic models.
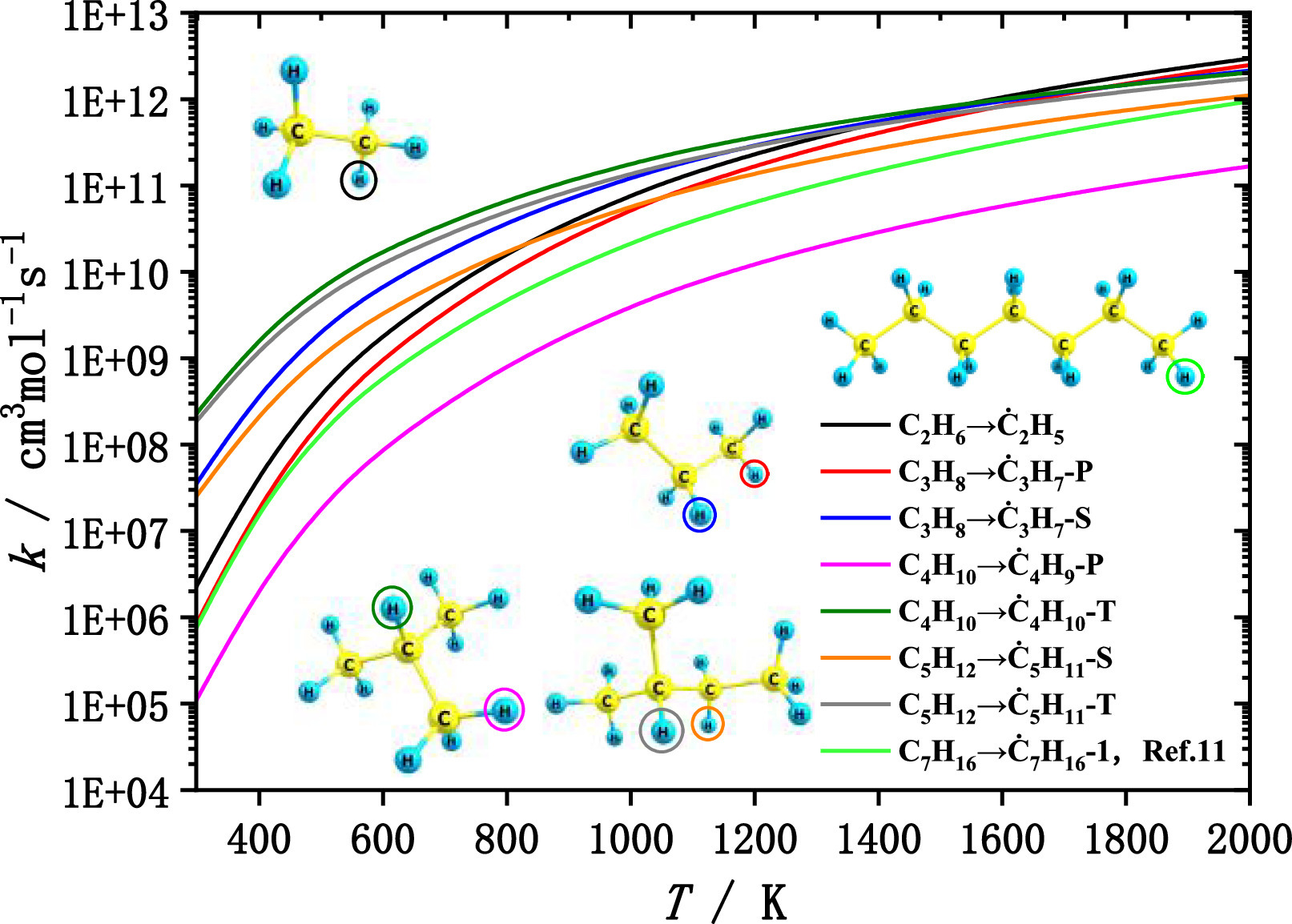
Fig. 2. Rate coefficients for alkanes + CH3Ȯ. (X. Ren, Y. Li*, et al. Combustion and Flame 263 (2024).)
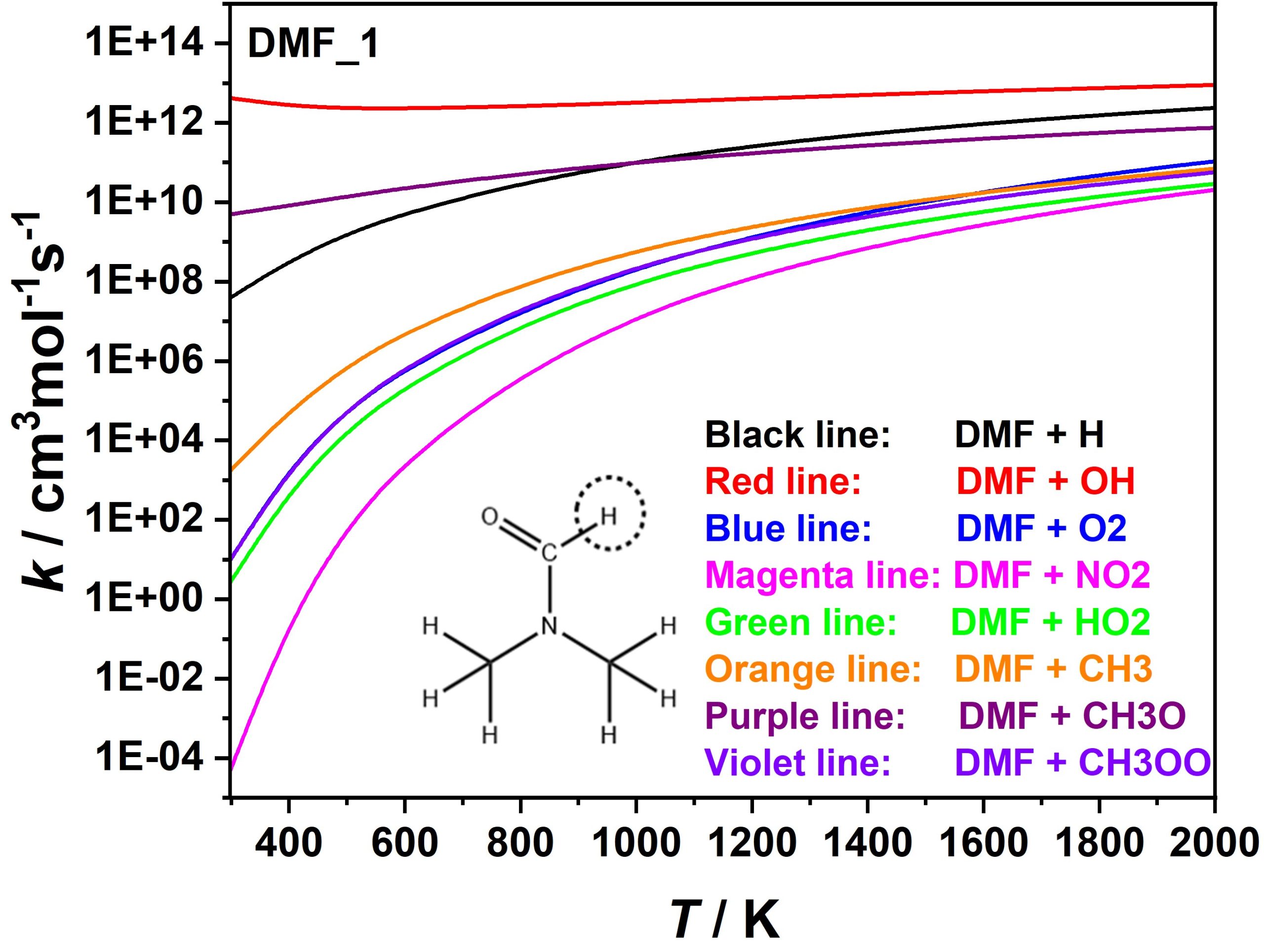
Fig. 3. Rate constants of H-atom abstraction from aldehyde group of DMF. (X. Bai, Y. Li*, et al. Fuel 353 (2023).)
Molecular dynamics simulation
MD simulation of energetic materials is another research field of our team. By constructing and simulating molecular systems in the decomposition and combustion processes of energetic materials, we analyze the intrinsic decomposition and combustion reaction pathways. These simulation results help to reveal the microscopic mechanisms of the reactions and assist us in supplementing and improving the reaction network of the kinetic model.
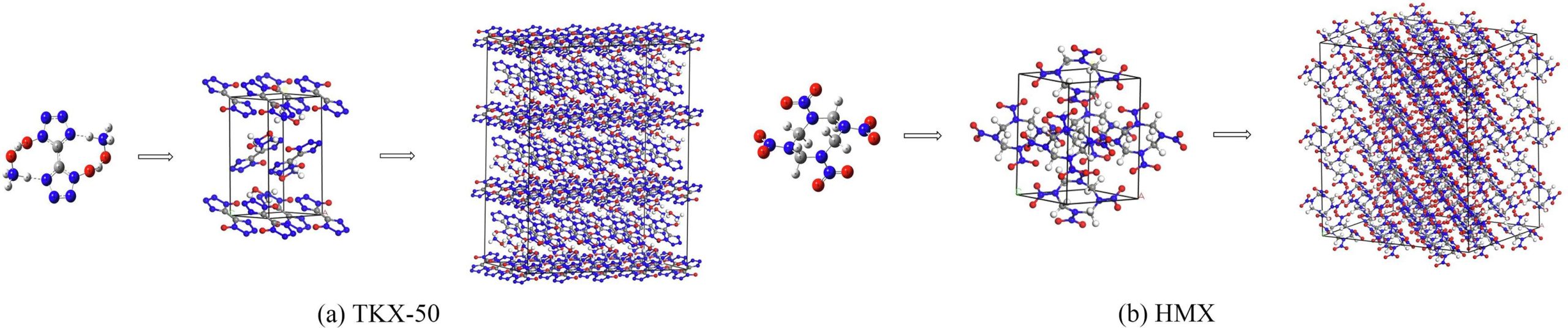
Fig. 4. Molecular structures, crystal lattices and supercells of (a) TKX-50 and (b) HMX. (X. Ren, Y. Li*, et al. Fuel 375 (2024) 132623.)
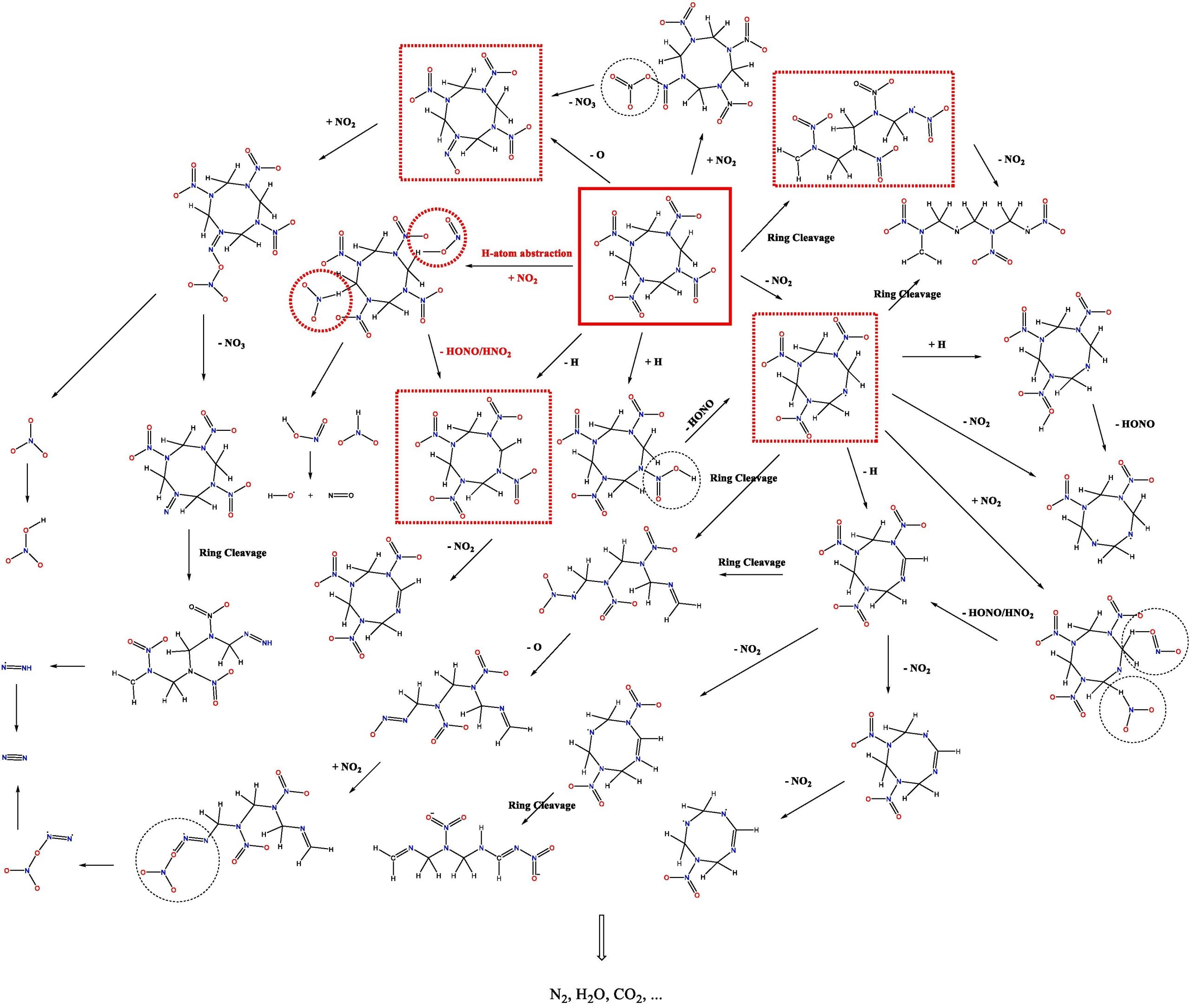
Fig. 5. Possible decomposition pathways of HMX. (X. Ren, Y. Li*, et al. Fuel 375 (2024) 132623.)
Machine Learning Prediction
Machine learning techniques show great potential in the field of reaction kinetics and thermochemical prediction. Our group builds models to learn complex patterns from large amounts of experimental data and theoretical calculations to predict rate constants (to be confirmed) and thermodynamic properties of chemical reactions.
Table 1. Model performance for enthalpy of formation at 298.15 K. (F.N.O. Bruce, Y. Li*, et al. Fuel 384 (2025).)
Feature-Method | Train_MAE (kcal/mol) | Test_MAE (kcal/mol) | Train_RMSE (kcal/mol) | Test_RMSE (kcal/mol) | Train Accuracy |
CDS-RF | 0.82 | 0.84 | 1.08 | 1.1 | 0.99 |
MRD-RF | 2.49 | 2.61 | 3.89 | 4.28 | 0.99 |
MBTR-RF | 6.09 | 6.26 | 4.43 | 4.62 | 0.97 |
MRD-XGB | 2.45 | 5.18 | 3.17 | 7.12 | 0.98 |
CDS-ANN-KR | 4.42 | 5.07 | 6.74 | 7.31 | 0.98 |
CM-RF | 5.19 | 5.39 | 7.15 | 7.37 | 0.97 |
CDS-XGB | 3.99 | 5.54 | 5.32 | 7.71 | 0.98 |
ECFP-RF | 6.65 | 6.7 | 8.77 | 8.52 | 0.96 |
SOAP-RF | 8.3 | 8.55 | 6.31 | 6.52 | 0.96 |
GNF-MPNN | 3.58 | 6.45 | 5.21 | 10.19 | 0.99 |
DRAG-RF | 7.79 | 8.2 | 11.69 | 12.22 | 0.93 |
ALVA-RF | 7.79 | 8.15 | 11.7 | 12.18 | 0.93 |
MRD-OCHEM-RF | 7.62 | 8.09 | 11.7 | 12.24 | 0.93 |
RDKIT-RF | 7.96 | 8.22 | 11.8 | 12.21 | 0.93 |
PYD-RF | 8.11 | 8.63 | 11.9 | 12.56 | 0.93 |
ACSF-RF | 9.51 | 9.35 | 11.92 | 12.00 | 0.94 |
2D-FIN-RF | 8.35 | 8.79 | 12.51 | 13.25 | 0.92 |
GNF-GCN | 8.96 | 9.30 | 12.56 | 13.15 | 1.00 |
GNF-GAT | 10.34 | 13.12 | 14.85 | 18.75 | 0.99 |
CDS-DT | 6.55 | 14.12 | 11.22 | 20.88 | 0.96 |
CDS-EXT | 27.24 | 29.41 | 34.67 | 36.4 | 0.83 |
ECFP-SVR | 17.08 | 44.89 | 17.95 | 57.48 | 0.86 |
MBTR-SVR | 17.34 | 47.39 | 18.1 | 59.3 | 0.85 |
MRD-SVR | 17.35 | 48.82 | 18.13 | 60.66 | 0.85 |
CM-SVR | 17.36 | 49.24 | 18.14 | 61.00 | 0.85 |
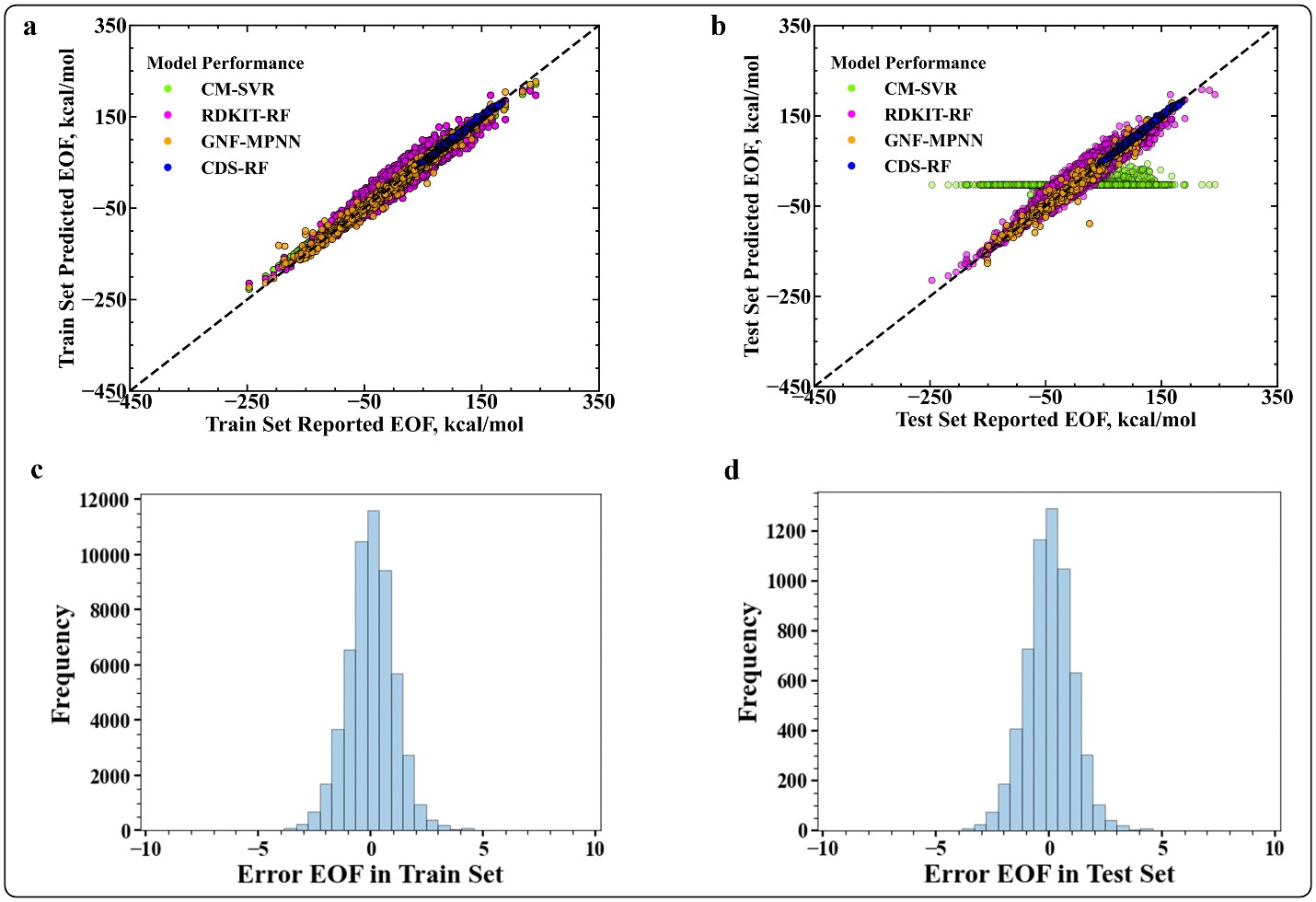
Fig. 6. Representative EOF performing models on the train (a) and test set (b). EOF error distribution in train (c) and test set (d) for the CDS-RF model. (F.N.O. Bruce, Y. Li*, et al. Fuel 384 (2025).)
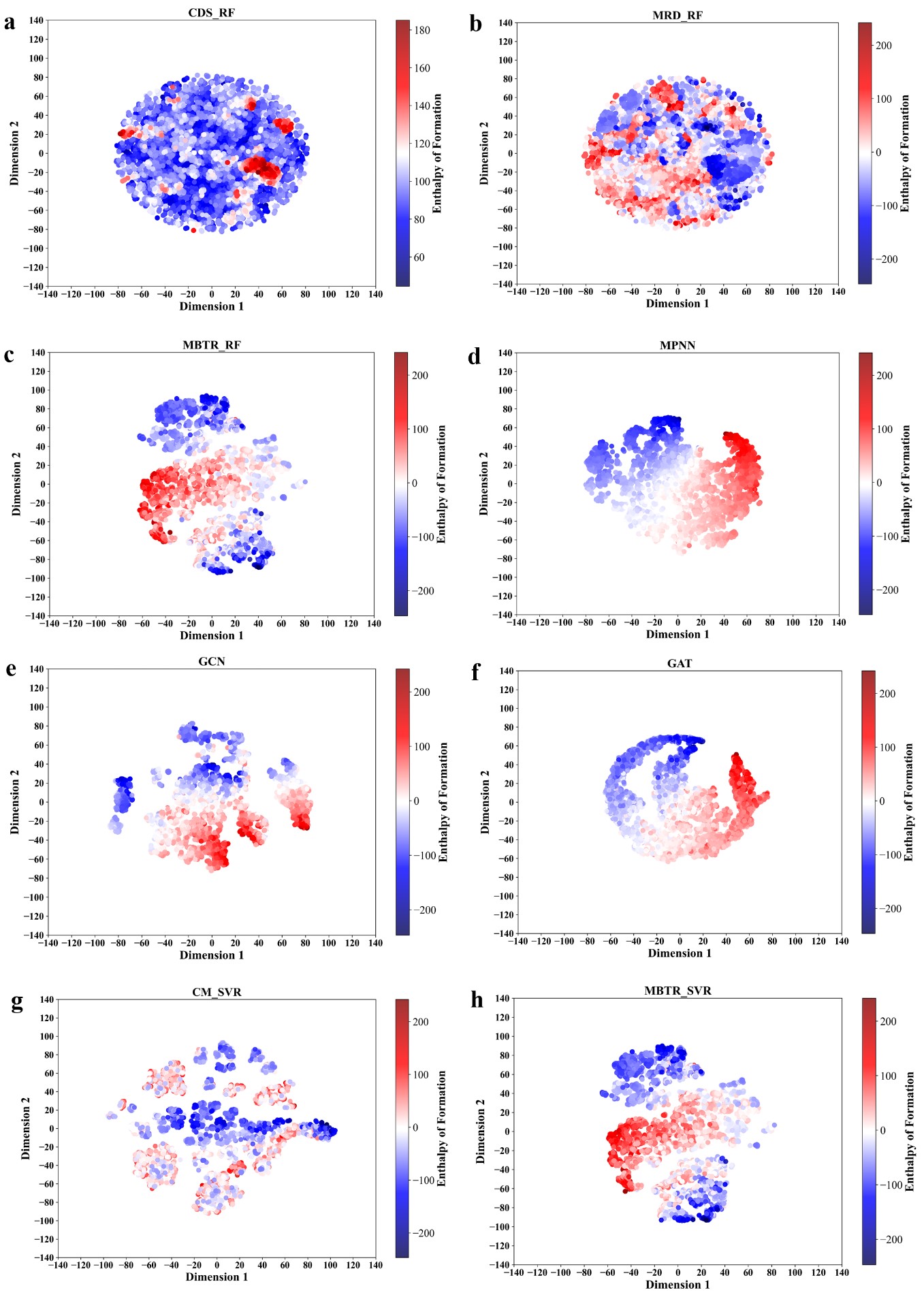
Fig. 7. t-SNE plots of Conventional and GNN models. (F.N.O. Bruce, Y. Li*, et al. Fuel 384 (2025).)